Digital Art Bias
Biometric Bias and the Creative Lens: Participation, Visibility & Ethics in Digital Art
Reflect on biometric bias and its creative implications. Learn what artists need to consider in tech and art environments.
Introduction
As it becomes more difficult to find ourselves able to seperate the physical from the digital, algorithms often serve as gatekeepers of identity and visibility. Biometric technologies like facial recognition and voice ID promise seamless authentication and novel creative tools – yet they also inherit human biases. Biometric bias refers to the systematic skew or errors in these systems that lead to unequal treatment or accuracy across different groups miteksystems.comadam.harvey.studio. For instance, it’s been shown that some commercial facial recognition algorithms frequently misclassify women with darker skin tones, sometimes even labeling famous Black women as male npr.org. Such examples reveal that biases in data and design can quite literally render certain people invisible or misrepresented in the eyes of technology.

An algorithm detects a mask as a face on a user it previously did not recognize – a striking demonstration of how biased facial recognition can fail to see certain faces until presented with a lighter mask npr.org.
This issue matters for everyone, but it carries special weight for digital artists and creative makers. Artists often engage with cutting-edge tech in their work, whether by using AI to generate visuals or by critiquing surveillance systems. If the underlying algorithms are biased, the creative outcomes and the audience’s experience can be distorted. Moreover, many contemporary artists are actively probing these biases, using art to expose how algorithmic classification can reinforce old stereotypes or create new forms of discrimination. Before diving deeper, let’s clarify what biometric bias entails and why it demands the attention of the creative community.
Understanding Biometric Bias
Biometric systems analyze physiological or behavioral traits – faces, fingerprints, voices, irises – to identify or verify individualsmiteksystems.com. Biometric bias occurs when these systems perform unevenly or unfairly across different populations. In essence, the algorithm that “sees” or compares biometric data ends up favoring certain faces or bodies over others, often due to imbalances in how it was trained or designed miteksystems.commiteksystems.com. A common cause is skewed training data: if a facial recognition model learns primarily from a dataset of light-skinned, male faces, it will excel at recognizing those faces but struggle with faces that deviate from that narrow profile. As one project on facial recognition notes, a “Euro-centric” dataset led to lower matching performance for underrepresented faces adam.harvey.studio. In plain terms, the software was much less accurate for people who weren’t white males, because it hadn’t really “learned” how to see them.
Crucially, biometric bias is not just a theoretical concern – it has been measured and documented.
A 2019 study of 189 face recognition algorithms found significantly higher error rates for women and people of color; in fact, African-American and Asian faces were 10 to 100 times more likely to be misidentified than Caucasian faces
miteksystems.com. Joy Buolamwini’s pioneering research at MIT Media Lab brought this issue to global attention when her test showed that systems from major tech companies mislabeled iconic Black women (like Michelle Obama and Serena Williams) as malenpr.org. Her accompanying artful video “AI, Ain’t I a Woman?” underscored these failings and prompted several companies to reassess their algorithmsnpr.org. These revelations drive home the point: biometric bias is real, and it reflects how social prejudices and blind spots can seep into our most advanced technologies.
Equally troubling is the resurgence of pseudoscientific ideas under the guise of AI. Some algorithms attempt to infer traits like trustworthiness, criminality, or emotional state just from a face – echoing the 19th-century debunked practice of physiognomy, which presumed one could judge character from facial features gradschool.designbynuff.com. Creative technologists have demonstrated how this can perpetuate bias. For example, an art installation titled “Biometric Bias” (2019) invited people to have their faces rated by an algorithm on attributes like attractiveness, “threat” level, and depression gradschool.designbynuff.com. The project was purposefully provocative, highlighting that such classifications are arbitrary and potentially prejudiced – a reminder that algorithms may inherit the flawed notions of their creators gradschool.designbynuff.com.
In short, biometric bias matters because it can encode societal biases into supposedly objective tools, making it an issue of fairness, accuracy, and human rights.
Participation and Visibility in Digital Systems
For artists and audiences, one immediate implication of biometric bias is how it affects who gets seen and who gets excluded in digital systems. A tool or artwork that relies on facial detection might simply not work for certain viewers. There have been infamous cases of technology literally failing to perceive people with darker skin – as in the viral video where a webcam’s face-tracking feature eagerly followed a white face but ignored a Black face entirelywired.com. The embarrassed manufacturer eventually admitted the system struggled in low-contrast lighting conditions, effectively rendering Black users invisible under typical home lightingwired.com. When such “computer vision” is embedded in art installations or apps, it raises serious issues of participation: if an interactive artwork doesn’t recognize a segment of the audience, those people are denied a full experience through no fault of their own.
Conversely, bias can also mean being hyper-visible in the worst ways. In security and surveillance contexts, biased biometrics can unfairly target certain groups. One study found that African Americans were disproportionately represented in police facial recognition databases (largely due to higher arrest rates and historical biases in policing), leading to more false matches and false suspicions against themmiteksystems.com. In other words, a biased system might “see” some people as threats more often simply because of skewed data. Artists concerned with social justice have noted this dynamic: technologies can either erase you (if you don’t fit the norm) or single you out (by over-scrutinizing you) – both are problematic for a healthy society.
From a creative standpoint, these issues of visibility cut two ways. Some artists respond by reclaiming invisibility as empowerment, devising ways to opt out of the machine’s gaze. For instance, artist Adam Harvey’s project CV Dazzle demonstrates avant-garde hair and makeup designs that confuse face-detection algorithmsen.wikipedia.orgen.wikipedia.org. By applying bold, asymmetric patterns of face paint and hairstyle, a person can slip under the radar of common computer vision systems. What Harvey discovered is striking: even a “nearly infallible” post-9/11 surveillance algorithm could be foiled by something as simple as makeup and a hairstyle adam.harvey.studio. This creative tactic flips the script on visibility – bias in the algorithm (its over-reliance on certain facial features) is exploited to achieve privacy. On the other hand, many artists aim to force visibility on the biases themselves. They build works that make the issue glaringly obvious, effectively holding a mirror up to the machine. Joy Buolamwini’s aforementioned performance did this by showing the absurd mis-gendering in real time, and it compelled viewers (and tech CEOs) to confront the uncomfortable truth npr.orgnpr.org. In either case, whether by hiding from biased eyes or exposing their flaws, artists are actively reshaping what it means to be “seen” in a digital world.
Ethics, Representation, and Algorithmic Bias
The prevalence of biometric bias brings a host of ethical questions to the forefront. If an algorithm consistently treats certain identities unfairly – viewing one group as less trustworthy or failing to recognize another group entirely – is it acceptable to use that algorithm in art or in society at large? Most would argue it’s not. Bias in AI systems raises issues of discrimination, consent, and accountability. Who is responsible when a “black box” model yields a racist or sexist outcomenews.artnet.com? The creators of these systems might not have intended to encode bias, yet intent matters little to those harmed by the results. As artist Trevor Paglen notes, letting machine learning systems categorize humans can “easily… go horribly wrong”news.artnet.com. If left unchecked, such classification risks reinforcing oppressive stereotypes under the veneer of algorithmic objectivity.
Artists working with biometric data must grapple with these ethical dimensions, often more directly than engineers do. Creative projects can surface questions about representation: Which faces or bodies are used to train an AI, and which are left out? Are the datasets themselves fair, or are they historically biased collections of images? In the landmark exhibition Training Humans (2019) by Kate Crawford (an AI scholar) and Trevor Paglen (an artist), the walls were plastered with thousands of images from real machine learning datasets – mugshots, selfies, passport photos – that have been used to “teach” algorithms how to recognize peoplenews.artnet.com. By curating these images in a gallery, the exhibition forced a reflection on how humans are represented, interpreted, and codified in the eyes of AI. Viewers could see patterns: the assumptions and prejudices embedded in the act of sorting people into categories. This kind of artwork makes the ethics tangible. It asks: Who gets to define the categories (male/female, healthy/sick, safe/dangerous), and what happens when those definitions are fed into powerful systems?
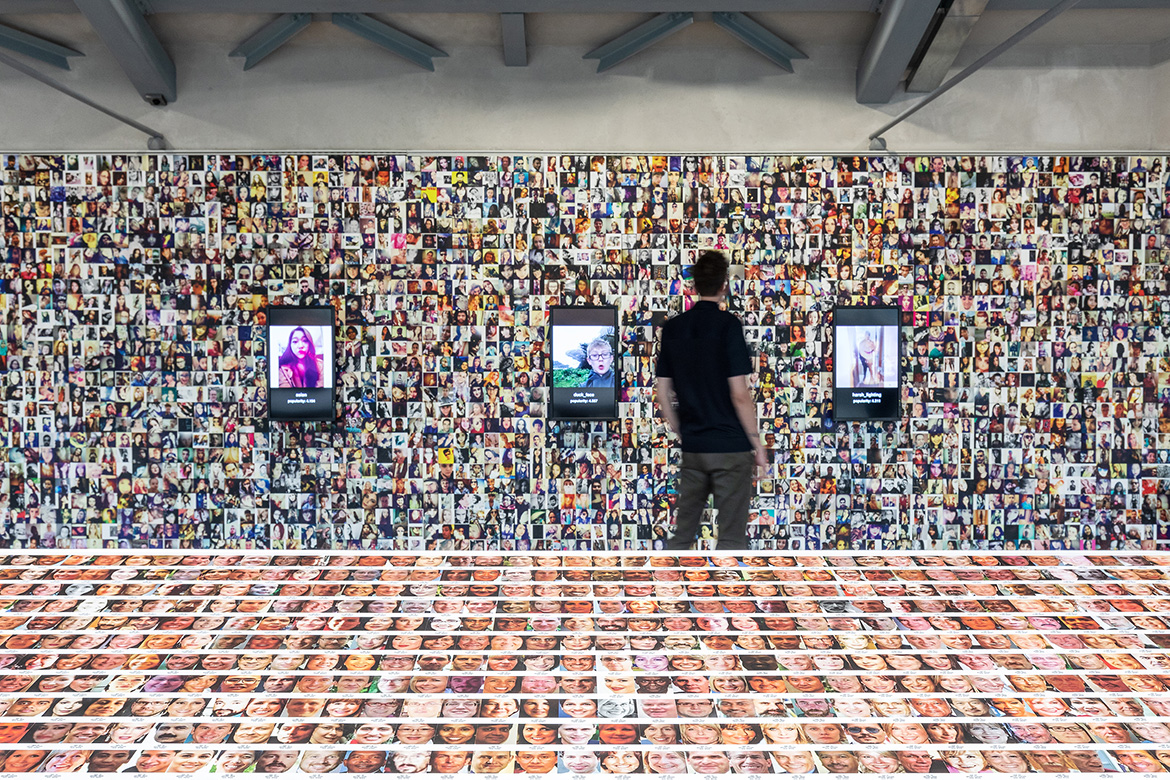
Exhibition view of Training Humans (2019) by Kate Crawford and Trevor Paglen, which displayed image datasets used to “recognize” people in AI systemsnews.artnet.comnews.artnet.com. Such projects reveal how biased or absurd the algorithm’s view of humanity can be.
Critically, many artists emphasize that biased algorithms don’t arise in a vacuum – they are extensions of long-standing social biases. By drawing parallels to historical practices like physiognomy or phrenology, creative works remind us that the desire to classify (and thereby control) people is not new, though it is now cloaked in codegradschool.designbynuff.com. This awareness leads to a call for ethical accountability. Some museums and festivals are now cautious about deploying facial recognition in their exhibits, precisely out of concern that it might treat visitors unequally or violate privacy. In academic circles, scholars advocate for “algorithmic audits” and inclusion of diverse perspectives when designing biometric systemsmiteksystems.comnpr.org. Interestingly, artists have been among the first to perform these audits in the public eye. By hacking, repurposing, or dramatizing AI systems, they reveal how the tech sees us – and whose reflections are missing or warped. This blend of ethics and aesthetics provides an accessible entry point for broader audiences to grasp why fairness in AI isn’t just a technical issue, but a cultural one.
Artistic Responses to Biometric Bias
A growing number of contemporary projects and artists explicitly tackle biometric bias, often with a critical or subversive edge. Below we highlight a few influential examples, each illuminating different facets of the issue:
Joy Buolamwini – AI, Ain’t I a Woman?: A MIT researcher-artist who uses poetry and video to expose facial recognition bias. In her viral spoken-word piece “AI, Ain’t I a Woman?”, Buolamwini dons a white mask to demonstrate how an algorithm fails to detect her dark-skinned face until she covers it with a pale surfacenpr.org. The title references Sojourner Truth’s famous abolitionist speech, linking past and present struggles for recognition. Buolamwini’s work (and the ensuing Coded Bias documentary) not only revealed that facial recognition misgenders women of colornpr.org, but also catalyzed a movement for algorithmic justice. She founded the Algorithmic Justice League to advocate for more equitable AI, proving art and activism can intersect to influence real-world tech ethicsnpr.org.
Trevor Paglen & Kate Crawford – ImageNet Roulette: This interactive online art project (2019) invited people to upload their photos and see how an AI trained on a popular dataset (ImageNet) would label themnews.artnet.com. The results ranged from amusing to deeply offensive. For example, the system tagged a Black man as “wrongdoer, offender” and an Asian woman as “Jihadist” based purely on their appearancenews.artnet.comnews.artnet.com. These shocking mislabels were not the AI “going rogue” – they exposed the systemic biases in the training data and category definitions. Paglen and Crawford used this viral experiment to demonstrate how prejudice can be codified in AI systems under the guise of objectivity. The project gained massive attention and even pressured the ImageNet database to remove over half a million problematic images and labelsnews.artnet.comnews.artnet.com. As Paglen noted, classifying humans is a dangerous endeavor – one that can easily replicate racist, sexist logics if we’re not carefulnews.artnet.com.
Adam Harvey – CV Dazzle and HyperFace: Adam Harvey is an artist known for creatively outsmarting surveillance AI. In his project CV Dazzle (2010–2013), he developed high-fashion makeup and hair styles that break facial recognition algorithms. By obscuring key facial features with bold designs, these looks exploit the algorithm’s expectations, causing it to fail to register a faceadam.harvey.studio. The concept’s success highlighted how fragile these systems can be – a reminder that what the computer deems important (symmetry, the bridge of the nose, eye contrast) can be subverted artisticallyadam.harvey.studioadam.harvey.studio. Harvey’s later project HyperFace (2016) extended this idea to textiles, creating patterned clothing that confuses computer vision by presenting false face-like signalstheguardian.com. These playful yet political works give individuals tools to reclaim privacy and comment on a world of ubiquitous surveillance. They turn the presence of bias (the system’s rigid focus on certain visual cues) into an opportunity for creative resistance.
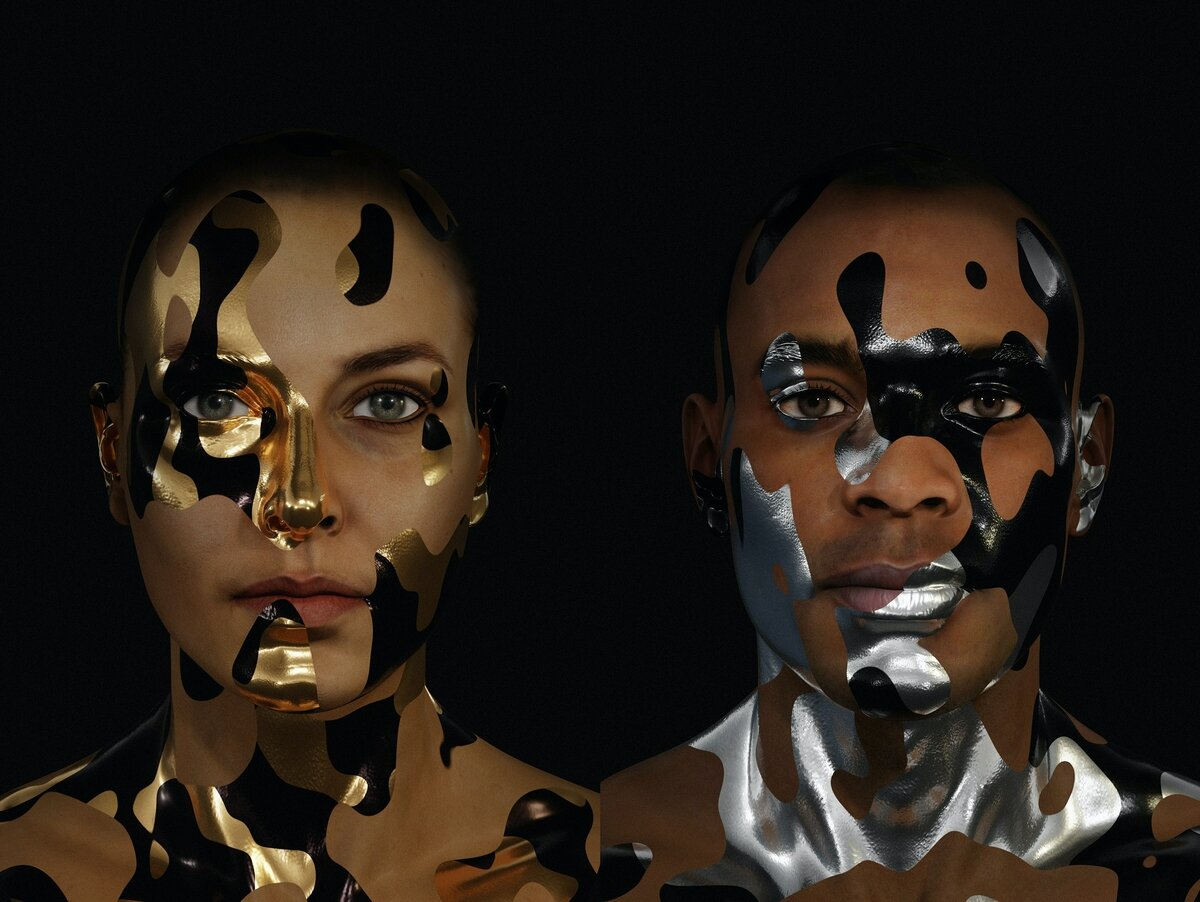
An example of CV Dazzle camouflage by Adam Harvey. By altering light/dark patterns on the face, the subject’s appearance falls below the threshold of detection for common face-recognition algorithms adam.harvey.studio. Artistic interventions like this reveal the mechanisms of biometric vision and how they can be subverted.
Zach Blas – Facial Weaponization Suite: Zach Blas, an artist and theorist, took a more confrontational approach to critiquing biometric systems. In Facial Weaponization Suite (2012–2014), Blas led community workshops to create “collective masks” – plastic masks formed by algorithmically merging the faces of multiple participants designmuseum.nl. The resulting mask is a featureless, amorphous visage that cannot be recognized as a human face by surveillance cameras designmuseum.nl. Each mask carried a political message. One, called the “Fag Face” mask, was generated from the faces of queer men as a protest against studies claiming to detect sexuality via face recognition designmuseum.nl. Another mask addressed race, highlighting “the inability of biometric technologies to detect dark skin” – a built-in bias that Blas frames as racist designmuseum.nl. By wearing these masks in public interventions, participants collectively embodied a refusal of legibility. Blas’s work exposes the inequalities perpetuated by biometric surveillance and suggests that obscuring oneself can be a form of solidarity and dissent.
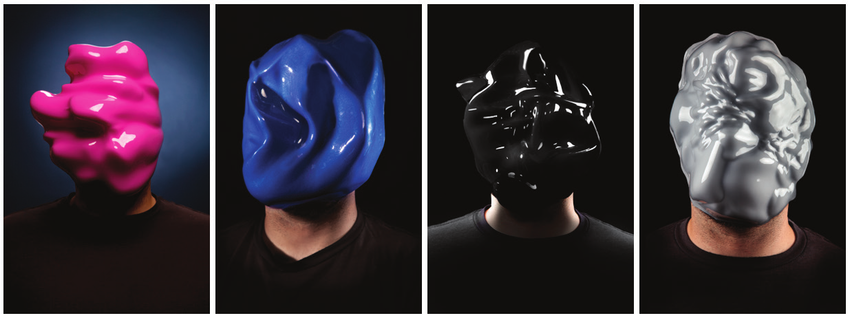
Zach Blas, Facial Weaponization Suite: Fag Face Mask, October 20, 2012, Los Angeles, CA, Mask, November 20, 2013, New York, NY, Mask, May 31, 2013, San Diego, CA, Mask, May 19, 2014, Mexico City, Mexico, photos by Christopher O’Leary.
Niels Wouters et al. – Biometric Mirror: A collaboration between artists and technologists, Biometric Mirror (2018) is an interactive installation that acts like a “smart” mirror – but with a twist. When you stand in front of it, an AI analyzes your face and tells you what it “sees”: your perceived age, mood, attractiveness, weirdness, etc. The catch is that these judgments are based on biased algorithms and questionable data correlations. The mirror might call someone “responsible” or “aggressive” looking, revealing more about cultural biases than about the person. By confronting participants with absurd or uncomfortable characterizations, Biometric Mirror provokes reflection on the ethics of AI judgmentcis.unimelb.edu.au. It essentially says: this is how algorithms might quietly judge us – do we really want that? The project underscores that such systems are far from neutral; they carry the prejudices of their makers and the flaws of their training data. Exhibited in science museums and galleries, Biometric Mirror straddles art and research, turning an AI funhouse into an educational commentary on the fallibility of algorithmic perceptioncis.unimelb.edu.aucis.unimelb.edu.au.
These examples (among many others) illustrate how artists are critically engaging with biometric bias. Whether through direct activism, satirical mimicry, or speculative scenario, each project makes the invisible visible. They translate abstract issues of data and bias into human terms – images, performances, and interactions one can feel. In doing so, they invite not just the art world but also technologists and the public to reckon with questions of who is reflected in our digital mirrors, and who is left out or distorted.
Looking Ahead: Speculative Inflections and Conclusions
Biometric bias is a pressing challenge today, but it also raises profound questions about the future. As biometric identification and algorithmic decision-making seep deeper into everyday life – from smart homes to smart cities – the stakes of bias will only grow. One can easily imagine near-future scenarios that artists and writers are already beginning to explore: What if emotion-recognition AI decides who gets hired, but it consistently misreads the faces of certain ethnic groups? What happens when augmented reality glasses overlay “profiles” on people we look at, and those profiles are skewed by biased data? These speculative cases highlight that unless we address bias now, we risk baking inequality into the technological infrastructure of tomorrow.
On a more hopeful note, the intersection of art and technology offers paths forward. Artists bring a critical, human-centered lens that can complement engineering approaches to fairness. They excel at posing the “what if?” questions and envisioning alternative designs. For instance, some creatives are prototyping inclusive datasets and algorithms as art projects – essentially showing how AI could be re-imagined with diversity and equity at the core. Others propose transparency and consent mechanisms, where people have more control over if and how their biometric data is used. Such ideas, while speculative, feed into real policy discussions and innovation in AI ethics.
In conclusion, reflecting on biometric bias reveals much about our society’s values and blind spots. For digital artists and creative makers, it’s a call to action to be mindful of the tools they use and the systems they critique. Biometric bias matters not only because of technical fairness, but because it touches on dignity, visibility, and power. Artists, with their blend of academic insight and imaginative flair, are uniquely positioned to translate these concerns into compelling narratives and experiences. By doing so, they help ensure that as technology progresses, it does so with a more inclusive vision – one where our digital mirrors reflect everyone fairly, and creative innovation goes hand in hand with ethical responsibility.
Blas, Z. (2012–2014). Facial Weaponization Suite [Art project]. http://www.zachblas.info/works/facial-weaponization-suite/
Buolamwini, J. (2018). AI, Ain’t I a Woman? [Video essay]. Algorithmic Justice League. https://www.ajl.org/videos
Buolamwini, J., & Gebru, T. (2018). Gender shades: Intersectional accuracy disparities in commercial gender classification. Proceedings of Machine Learning Research, 81, 1–15. https://proceedings.mlr.press/v81/buolamwini18a.html
Crawford, K., & Paglen, T. (2019). Training Humans [Exhibition]. Fondazione Prada. https://www.fondazioneprada.org/project/training-humans/
Crawford, K., & Paglen, T. (2019). ImageNet Roulette [Interactive web project]. https://www.imagelabelling.net
Harvey, A. (2010–2013). CV Dazzle [Art project]. https://cvdazzle.com/
Harvey, A. (2016). HyperFace [Art project]. https://ahprojects.com/projects/hyperface/
Klare, B. F., Burge, M. J., Klontz, J. C., Vorder Bruegge, R. W., & Jain, A. K. (2012). Face recognition performance: Role of demographic information. IEEE Transactions on Information Forensics and Security, 7(6), 1789–1801. https://doi.org/10.1109/TIFS.2012.2214212
Raji, I. D., & Buolamwini, J. (2019). Actionable auditing: Investigating the impact of publicly naming biased performance results of commercial AI products. Proceedings of the 2019 AAAI/ACM Conference on AI, Ethics, and Society, 429–435. https://doi.org/10.1145/3306618.3314244
Wouters, N., Hasan, M., Morrison, A., Vande Moere, A., & Leahu, L. (2018). Biometric Mirror: Exploring ethical implications of AI in commercial facial analysis applications. Proceedings of the 2018 ACM Conference on Human Factors in Computing Systems (CHI). https://doi.org/10.1145/3173574.3174027